Effective Data Cleaning Techniques: Guide
You’re drowning in data, but is it all useful? Often, it’s not. Your data needs a good scrub to shine. That’s where data-cleaning techniques come into play.
In this article, we’ll explore how to:
- Remove duplicates
- Handle missing values
- Standardize and normalize values
- Manage outliers
Let’s dive right in and learn how to transform messy data into a goldmine of insights.
Table of Contents
Understanding the Importance of Quality Information
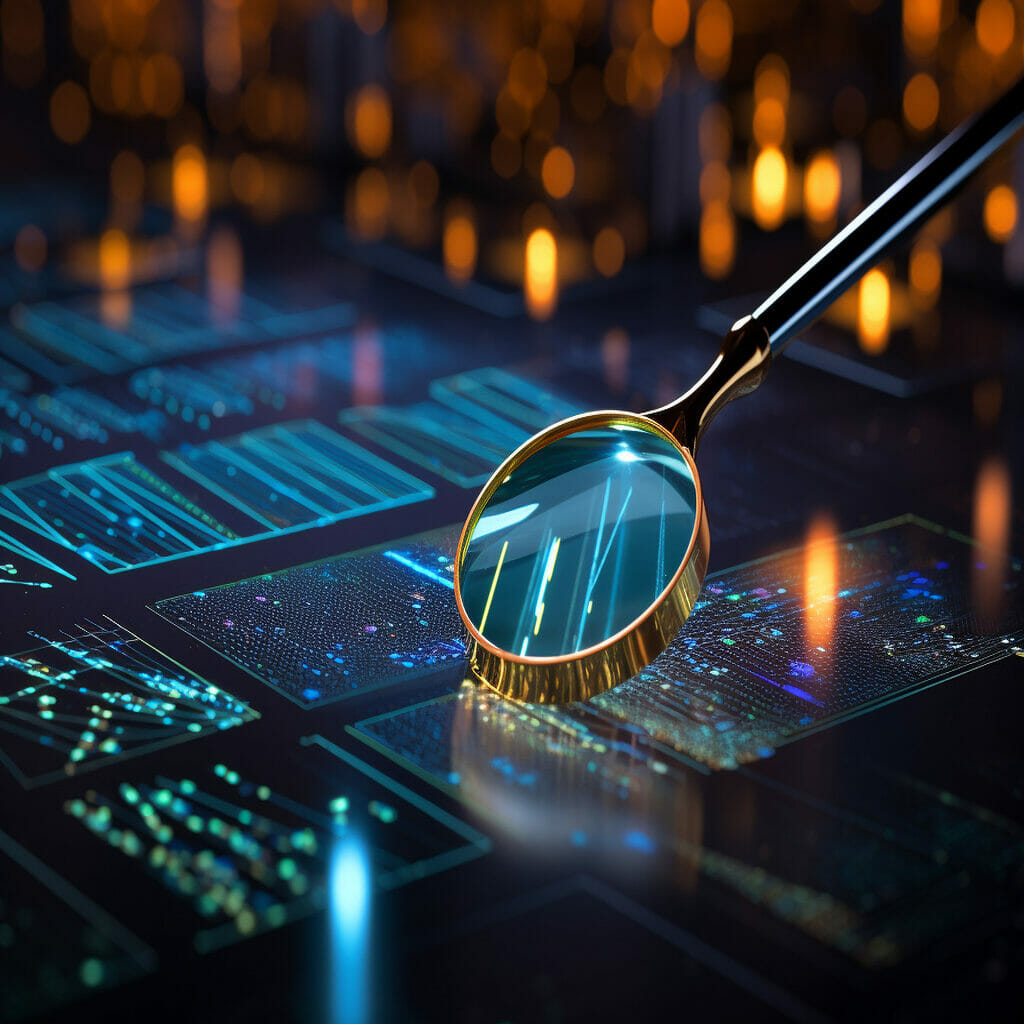
You’ve got to understand the importance of quality information. It’s the foundation for reliable analysis and decision-making in data cleaning techniques.
Picture this: your data is a house. If it’s built with faulty materials, it’s bound to crumble. In the same way, data analysis and decision-making will only succeed if based on accurate data.
Data cleaning enhances the quality of your information, making it trustworthy and reliable. It’s about correcting errors, inconsistencies, or inaccuracies that creep into your data sets. It’s also about removing duplicates and filling in missing values.
So, you see, data cleaning isn’t just about tidying up; it’s about reinforcing the integrity of your data. It’s vital to ensure your data is solid, reliable, and valuable for your business decisions.
Removing Duplicate Entries
To maintain the integrity of your analysis, it’s crucial to eliminate duplicate entries. These duplicates can skew your results and provide an inaccurate representation of the data. There are various techniques to handle this issue.
A straightforward method is deduplication, where you systematically identify and remove exact duplicate entries.
Another technique is fuzzy matching, which is useful when the duplicates aren’t exact but very similar. This method uses algorithms to identify entries likely duplicates based on specific parameters.
However, be careful not to eliminate data that may seem duplicate but are unique entries. Always double-check and validate your data cleaning process.
Remember, accurate and clean data is the foundation for successful data analysis.
Handling Missing Values
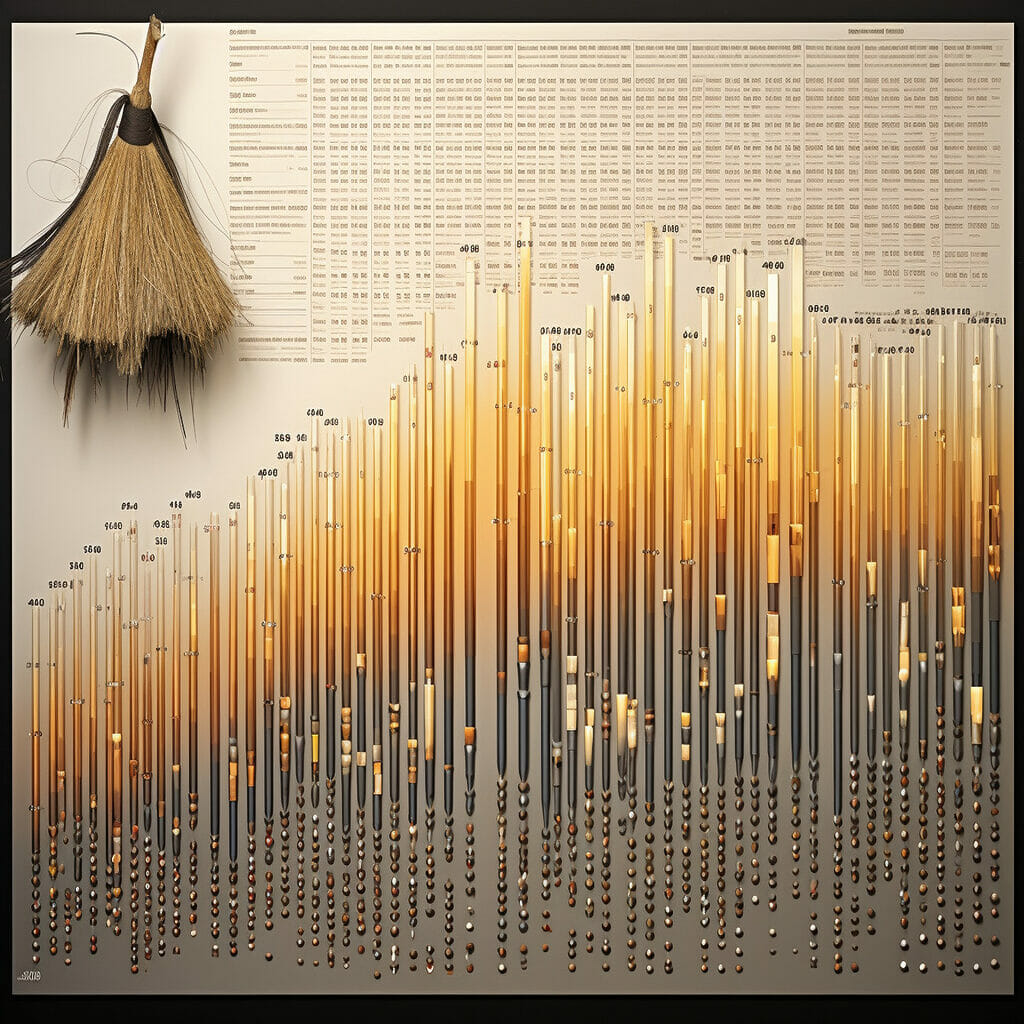
Dealing with missing values is another crucial step during your analysis. Sometimes, your dataset may have lost or incomplete entries. Handling these appropriately is essential, as they can significantly distort your analysis results.
There are several ways to deal with missing data. First, you can ignore them, but this is not usually recommended unless the missing data is random or insignificant.
Second, you can delete the rows with missing values, which can be helpful if the missing data is substantial and randomly distributed.
Third, you can fill the missing values with a specific value like zero, mean, median, or mode.
Lastly, advanced regression or machine learning algorithms can predict and fill in missing values.
Standardizing and Normalizing Values
Next, let’s focus on standardizing and normalizing your values, which is critical to ensure comparability and construct reliable models.
When you standardize data, you adjust its scale so that the values have a mean of zero and a standard deviation of one. This way, you can compare features that have different units or scales.
Conversely, normalizing adjusts your data to a standard scale, typically between 0 and 1. This is useful when dealing with parameters of different scales, and we don’t want any of them to dominate the model due to their large numbers.
By using these techniques, you’re ensuring that your data is ready for further analysis and model building, enhancing the accuracy of your results.
Outlier Detection and Management
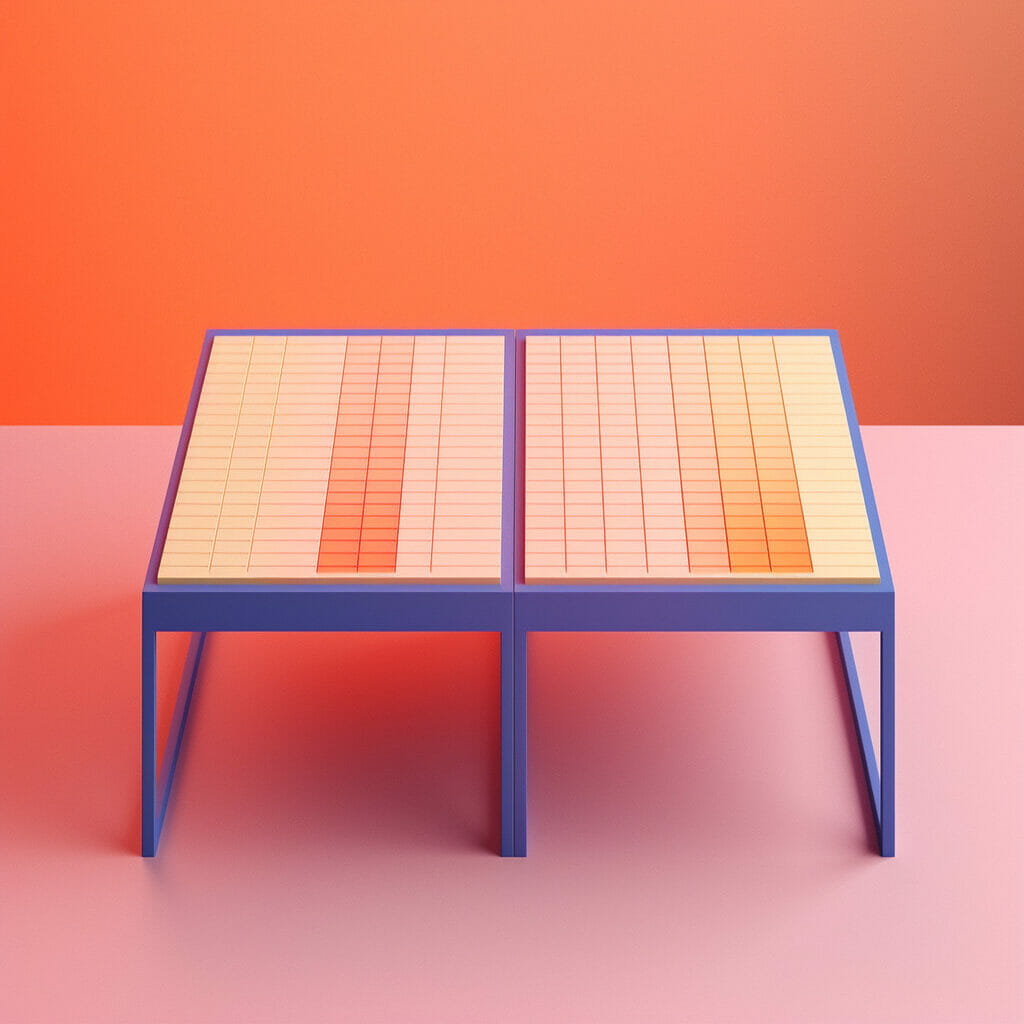
Managing outliers effectively is crucial as they can significantly skew your model’s results. Outliers are data points that deviate significantly from other observations. They can be caused by variability in the data or possible errors.
The first step in managing outliers is detection. To identify potential outliers, you can visualize the data using box plots, scatter plots, or histograms. Statistical tests such as the Z-score or IQR method can also be used.
Once detected, you’ve got several options. If an outlier is due to a data entry error, you might correct or remove it from the dataset. You could keep it or apply transformation techniques to lessen its impact if it’s a legitimate data point. Remember, each outlier must be considered individually and handled with care.
Frequently Asked Questions
What is data cleaning?
Data cleaning, also known as data cleansing, identifies and corrects or removes errors, inconsistencies, and inaccuracies in datasets to improve data quality and ensure accurate and reliable data analysis.
What are the benefits of data cleaning?
The benefits of data cleaning include improved data accuracy and reliability, enhanced data quality, reduced errors and inconsistencies, increased efficiency in data analysis, better decision-making, and improved machine learning results.
What are some data-cleaning techniques?
Some standard data cleaning techniques include handling missing values, removing duplicate data, fixing inaccurate or corrupt data, standardizing and transforming data, dealing with outliers, and handling irrelevant or inconsistent data.
How does data cleaning relate to data mining?
Data cleaning is a crucial step in the data mining process. Cleaning and preprocessing the data ensures that the data used for data mining is accurate, complete, consistent, and suitable for analysis. Clean data is essential for obtaining meaningful and reliable insights from data mining.
What is the role of data quality in data cleaning?
Data quality refers to the degree to which data meets the requirements and standards of its intended use. Data cleaning aims to improve data quality by identifying and addressing data errors, inconsistencies, and inaccuracies, ensuring that the data type meets the desired level of quality for analysis.
Conclusion
In conclusion, you’ve seen how crucial quality information is. You’ve learned to eradicate duplicate entries, manage missing values, standardize and normalize, and handle outliers.
Apply these data-cleaning techniques and watch your data analysis improve. Quality data leads to quality decisions, so keep cleaning your data source.
It’s a constant journey but one well worth the effort.